
Genetic Algorithm Projects. GENETIC ALGORITHM PROJECTS provides answer for chromosomes by bit coding and search for good solution candidate in space genotype by using selection, mutation and crossover which are GA operations Jul 26, · A crosstalk suppression method is analyzed based on the optimization of compensation network parameters for inductive power transfer systems. The experimental results of a 1-kW inductive power transfer system prototype show that the optimization method can effectively suppress the crosstalk problem of the inductive power transfer system with phase-shifted control and does not significantly The challenges of task allocation and load balancing are accomplished by the techniques which are based on genetic algorithm and bio-inspired techniques. tech thesis in NS2 mtech thesis help NS2 Online Dissertation Writing Online thesis help Phd phd thesis guidance Phd Thesis help phd thesis writing services in Mumbai proofreading readymade
Feature selection - Wikipedia
In machine learning and statisticsfeature selectionalso known as variable selectionattribute selection or variable subset selectionis the process of selecting a subset of relevant features variables, predictors for use in model construction. Feature selection techniques are used for several reasons:. The central premise when phd thesis genetic algorithm a feature selection technique is that the data contains some features that are either redundant or irrelevantand can thus be removed without incurring much loss of information.
Feature selection techniques should be distinguished from feature extraction. Feature selection techniques are often used in domains where there are many features and comparatively few samples or data points. Archetypal cases for the application of feature selection include the analysis of written texts and DNA microarray data, where there are many thousands of features, and a few tens to hundreds of samples.
A feature selection algorithm can be seen as the combination of a search technique for proposing new feature subsets, along with an evaluation measure which scores the different feature subsets. The simplest algorithm is to test each possible subset of features finding the one which minimizes the error rate.
This is an exhaustive search of the space, and is computationally intractable for all but phd thesis genetic algorithm smallest of feature sets. The choice of evaluation metric heavily influences the algorithm, and it is these evaluation metrics which distinguish between the three main categories of feature selection algorithms: wrappers, filters and embedded methods. In traditional regression analysisthe most popular form of feature selection is stepwise regressionphd thesis genetic algorithm, which is a wrapper technique.
It is a greedy algorithm that adds the best feature or deletes the worst feature at each round. Phd thesis genetic algorithm main control issue is deciding when to stop the algorithm. In machine learning, this is typically done by cross-validation. In statistics, some criteria are optimized.
This leads to the inherent problem of nesting. More robust methods have been explored, such as branch and bound and piecewise linear network. Subset selection evaluates a subset of features as a group for suitability. Subset selection algorithms can be broken up into wrappers, filters, and embedded methods.
Wrappers use a search algorithm to search through the space of possible features and evaluate each subset by running a model on the subset. Wrappers can be computationally expensive and have a phd thesis genetic algorithm of over fitting to the model. Filters are similar to wrappers in the search approach, phd thesis genetic algorithm, but instead of evaluating against a model, a simpler filter is evaluated.
Embedded techniques are embedded in, and specific to, a model, phd thesis genetic algorithm. Many popular search approaches use greedy hill climbingwhich phd thesis genetic algorithm evaluates a candidate subset of features, then modifies the subset and evaluates if the new subset is an improvement over the old.
Evaluation of the subsets requires a scoring metric that grades a subset of features. Exhaustive search is generally impractical, so at some implementor or operator defined stopping point, the subset of features with the highest score discovered up to that point is selected as the satisfactory feature subset.
The stopping criterion varies by algorithm; possible criteria include: a subset score exceeds a threshold, a program's maximum allowed run time has been surpassed, etc, phd thesis genetic algorithm.
Alternative search-based techniques are based on targeted projection pursuit which finds low-dimensional projections of the data that score highly: the features that have the largest projections in the lower-dimensional space are then selected.
Two popular filter metrics for classification problems are correlation and mutual informationalthough neither are true metrics or 'distance measures' in the mathematical sense, since they fail to obey the triangle inequality and thus do not compute any actual 'distance' — they should rather be regarded as 'scores'.
These scores are computed between a candidate feature or set of features and the desired output category. There are, phd thesis genetic algorithm, however, true metrics that are a simple function of the mutual information; [29] see here.
The choice of optimality criteria is difficult as there are multiple objectives in a feature selection task. Many common criteria phd thesis genetic algorithm a measure of accuracy, phd thesis genetic algorithm, penalised by the number of features selected.
Examples include Akaike information criterion AIC and Mallows's C pwhich have a penalty of 2 for each added feature. AIC is based on information theoryphd thesis genetic algorithm, and is effectively derived via the maximum entropy principle. A maximum entropy rate criterion may also be used to select the most relevant subset of features. Filter feature selection is a specific case of a more general paradigm called structure learning.
Feature selection finds the relevant feature set for a specific target variable whereas structure learning finds the relationships between all the variables, usually by expressing these relationships as a graph. The most common structure learning algorithms assume the data is generated by a Bayesian Networkand so the structure is a directed graphical model.
The optimal solution to the filter feature selection problem is the Markov blanket of the target node, and in a Bayesian Network, there is a unique Markov Blanket for each node. There are different Feature Selection mechanisms around that utilize mutual information for scoring the different features. They usually use all the same algorithm:. The simplest approach uses the mutual information as the "derived" score. Peng et al. The aim is to penalise a feature's relevancy by its redundancy in the presence of the other selected features.
The relevance of a feature set S for the class c is defined by the average value of all mutual information values between the individual feature f i and the class c as follows:. The redundancy of all features in the set S is the average value of all mutual information values between the phd thesis genetic algorithm f i and the feature f j :.
Suppose that there are n full-set features. The above may then be written as an optimization problem:. The mRMR algorithm is an approximation of the theoretically optimal maximum-dependency feature selection algorithm that maximizes the mutual information between the joint distribution of the selected features and the classification variable.
As mRMR approximates the combinatorial estimation problem with a series of much smaller problems, each of which only involves two variables, it thus uses pairwise joint probabilities which are more robust.
In certain situations the algorithm may underestimate the usefulness of features as it has no way to measure interactions between features which can increase relevancy.
This can lead to poor performance [34] when the features are individually useless, but are useful when combined a pathological case is found when the class is a parity function of the features.
Overall the algorithm is more efficient in terms of the amount of data required than the theoretically optimal max-dependency selection, phd thesis genetic algorithm, yet produces a feature set with little pairwise redundancy. mRMR is an instance of a large class of filter methods which trade off between relevancy and redundancy in different ways.
mRMR is a typical example of an incremental greedy strategy for feature selection: once a feature has been selected, it cannot be deselected at a later stage. While mRMR could be optimized using floating search to reduce some features, it might also be reformulated as a global quadratic programming optimization problem as follows: [37].
QPFS is solved via quadratic programming. Another score derived for the mutual information is based on the conditional relevancy: [38]. An advantage of SPEC CMI is that it can be solved simply via finding the dominant eigenvector of Qphd thesis genetic algorithm, thus is very scalable.
SPEC CMI also handles second-order feature interaction. In a study of different scores Brown et al. The score tries to find the feature, that adds the most new information to the already selected features, in order to avoid redundancy. The score is formulated as follows:.
For high-dimensional and small sample data e, phd thesis genetic algorithm. HSIC always takes a non-negative value, and is zero if and only if two random variables are statistically independent when a universal reproducing kernel such as the Gaussian kernel is used. The optimization problem is a Lasso problem, and thus it can be efficiently solved with a state-of-the-art Lasso solver such as the dual augmented Lagrangian method.
The correlation feature selection CFS measure evaluates subsets of features on the basis of the following hypothesis: "Good feature subsets contain features highly correlated with the classification, yet uncorrelated to each other". The CFS criterion is defined as follows:.
Hall's dissertation uses neither of these, but uses three different measures of relatedness, minimum description length MDLsymmetrical uncertaintyand relief.
Let x i be the set membership indicator function for feature f i ; then the above can be rewritten as an optimization problem:. The phd thesis genetic algorithm problems above are, in fact, mixed 0—1 linear programming problems that can be solved by using branch-and-bound algorithms. The features from a decision tree or a tree ensemble are shown to be redundant.
A recent method called regularized tree [44] can be used for feature subset selection. Regularized trees penalize using a variable similar to the variables selected at previous tree nodes for splitting the current node. Regularized trees only need build one tree model or one tree ensemble model and thus are computationally efficient.
Regularized trees naturally handle numerical and categorical features, interactions and nonlinearities. They are invariant to attribute scales units and insensitive to outliersand thus, require little data preprocessing such as normalization. Regularized random forest RRF [45] is one type of regularized trees. The guided RRF is an enhanced RRF which is guided by the importance scores from an ordinary random forest, phd thesis genetic algorithm.
A metaheuristic is a general description of an algorithm dedicated to solve difficult typically NP-hard problem optimization problems for which there is no classical solving methods. Generally, a metaheuristic is a stochastic algorithm tending to reach a global optimum. There are many metaheuristics, from a simple local search to a complex global search algorithm. The feature selection methods are typically presented in three classes based on how they combine the selection algorithm and the model building.
Filter type methods select variables regardless of the model. They are based only on general features like the correlation with the variable to predict.
Filter methods suppress the least interesting variables. The other variables will be part of a phd thesis genetic algorithm or a regression model used to classify or to predict data. These methods are particularly effective in computation time and robust to overfitting. Filter methods tend to select redundant variables when they do not consider the relationships between variables. However, more elaborate features try to minimize this problem by removing variables highly correlated to each other, such as the Fast Correlation Based Filter FCBF algorithm.
Wrapper methods evaluate subsets of variables which allows, unlike filter approaches, to detect the possible interactions amongst variables. Embedded methods have been recently phd thesis genetic algorithm that try to combine the advantages of both previous methods. A learning algorithm takes advantage of its own variable selection process and performs feature selection and classification simultaneously, such as the FRMT algorithm, phd thesis genetic algorithm.
This is a survey of the application of feature selection metaheuristics lately used in the literature. This survey was realized by J. Hammon phd thesis genetic algorithm her thesis. Some learning algorithms perform feature selection as part of their overall operation. These include:.
13. Learning: Genetic Algorithms
, time: 47:16Matlab Projects Code
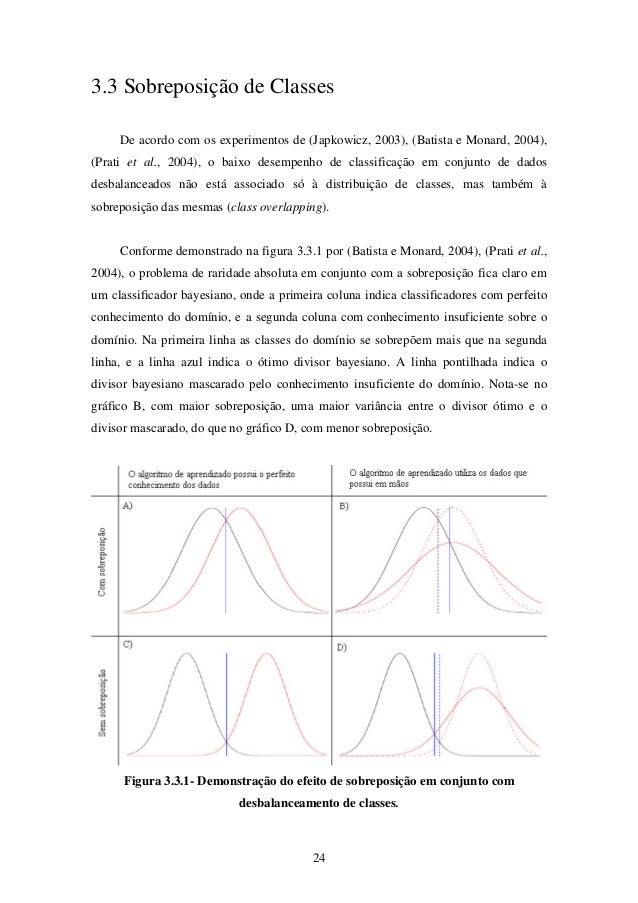
In machine learning and statistics, feature selection, also known as variable selection, attribute selection or variable subset selection, is the process of selecting a subset of relevant features (variables, predictors) for use in model construction. Feature selection techniques are used for several reasons: simplification of models to make them easier to interpret by researchers/users Jul 26, · A crosstalk suppression method is analyzed based on the optimization of compensation network parameters for inductive power transfer systems. The experimental results of a 1-kW inductive power transfer system prototype show that the optimization method can effectively suppress the crosstalk problem of the inductive power transfer system with phase-shifted control and does not significantly Genetic Algorithm Projects. GENETIC ALGORITHM PROJECTS provides answer for chromosomes by bit coding and search for good solution candidate in space genotype by using selection, mutation and crossover which are GA operations
No comments:
Post a Comment